Reference Class Forecasting
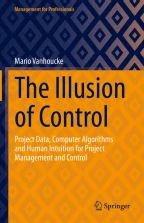
This chapter summarises the results of three studies using the reference class forecasting technique on empirical project data. Each project control method from the previous chapters focused on forecasting the time and costs of ongoing projects but rarely used past historical projects. The reference class forecasting is specially designed for estimating a project’s expected time and costs by explicitly taking project-specific characteristics of historical project data into account. It will be shown that this technique yields promising results and can therefore be perfectly combined with some methods from the previous chapters. Such a hybrid method can significantly increase the overall forecasting accuracy of projects.
This is a preview of subscription content, log in via an institution to check access.
Subscribe and save
Springer+ Basic
€32.70 /Month
- Get 10 units per month
- Download Article/Chapter or eBook
- 1 Unit = 1 Article or 1 Chapter
- Cancel anytime
Buy Now
Price includes VAT (France)
eBook EUR 85.59 Price includes VAT (France)
Softcover Book EUR 73.84 Price includes VAT (France)
Hardcover Book EUR 105.49 Price includes VAT (France)
Tax calculation will be finalised at checkout
Purchases are for personal use only
Notes
I must admit that I rarely read novels, because in the scientific literature, especially in the popular science literature, there can be so much excitement that it not only thoroughly improves my knowledge but also sometimes completely blows my imagination.
The study was published in Batselier and Vanhoucke (2016) and is a collaboration with Jordy Batselier, a PhD student at OR&S between 2012 and 2016 whom I will introduce in Chap. 10.
\(SPI(t)_\) is the instantaneous \(SPI(t)\) , reflecting the schedule performance over the last tracking interval. More specifically, \(SPI(t)_\) is calculated by dividing the increase in ES during the last tracking interval by the corresponding increment of AT, or \((ES_t - ES_)/(AT_t - AT_)\) . Notice the difference between \(SPI(t)_\) and the standard cumulative \(SPI(t)\) , which represents the schedule performance over the entire project up to the current tracking period.
For more information about the concepts of project authenticity and tracking authenticity for empirical project data, the readers are referred to Chap. 10.
I should really pay more attention to Tom’s work, as I did in Chap. 8 to Annelies’ work, but his research topic (project scheduling with alternative technologies) is somewhat beyond the scope of this book. I am sure I will write another book 1 day where I will describe his results in more detail, and I will refer to Tom again in Chap. 15.
A few weeks before I had sent my final version of this book to my publisher, I noticed that Bent Flyvbjerg’s new book had just come out. I immediately bought and read it, and I have no hesitation in recommending it to anyone interested in project management (Flyvbjerg & Gardner, 2023). Needless to say how pleased I was that our three studies on reference class forecasting are included in the reference list.
References
- Batselier, J., & Vanhoucke, M. (2015a). Construction and evaluation framework for a real-life project database. International Journal of Project Management, 33, 697–710. ArticleGoogle Scholar
- Batselier, J., & Vanhoucke, M. (2015b). Empirical evaluation of earned value management forecasting accuracy for time and cost. Journal of Construction Engineering and Management, 141(11), 1–13. ArticleGoogle Scholar
- Batselier, J., & Vanhoucke, M. (2016). Practical application and empirical evaluation of reference class forecasting for project management. Project Management Journal, 47(5), 36–51. ArticleGoogle Scholar
- Batselier, J., & Vanhoucke, M. (2017). Improving project forecast accuracy by integrating earned value management with exponential smoothing and reference class forecasting. International Journal of Project Management, 35, 28–43. ArticleGoogle Scholar
- Cantarelli, C., & Flyvbjerg, B. (2015). Decision making and major transport infrastructure projects: The role of project ownership. In R. Hickman, M. Givoni, D. Bonilla, & D. Banister (Eds.), Handbook on Transport and Development (pp. 380–393). Edward Elgar Publishing. Google Scholar
- Cantarelli, C., Flyvbjerg, B., & Buhl, S. L. (2012a). Geographical variation in project cost performance: The Netherlands versus worldwide. Journal of Transport Geography, 24, 324–331. ArticleGoogle Scholar
- Cantarelli, C., Molin, E., van Wee, B., & Flyvbjerg, B. (2012b). Characteristics of cost overruns for Dutch transport infrastructure projects and the importance of the decision to build and project phases. Transport Policy, 22, 49–56. ArticleGoogle Scholar
- Cantarelli, C., van Wee, B., Molin, E., & Flyvbjerg, B. (2012c). Different cost performance: Different determinants? the case of cost overruns in Dutch transport infrastructure projects. Transport Policy, 22, 88–95. ArticleGoogle Scholar
- Flyvbjerg, B., & Gardner, D. (2023). How big things get done: The surprising factors that determine the fate of every project, from home renovations to space exploration and everything in between. McClelland and Stewart. Google Scholar
- Flyvbjerg, B., Glenting, C., & Rønnest, A. (2004). Procedures for dealing with optimism bias in transport planning. London: The British Department for Transport, Guidance Document. Google Scholar
- Flyvbjerg, B., Skamris, M., & Buhl, S. (2002). Underestimating costs in public works projects: Error or lie? Journal of the American Planning Association, 68, 279–295. ArticleGoogle Scholar
- Kahneman, D. (2011). Thinking, fast and slow. New York: Farrar, Straus and Giroux. Google Scholar
- Kahneman, D., & Tversky, A. (1979a). Intuitive prediction: Biases and corrective procedures. In S. Makridakis & S. Wheelwright (Eds.), Studies in the management sciences: Forecasting (p. 12). Amsterdam: North Holland. Google Scholar
- Kahneman, D., & Tversky, A. (1979b). Prospect theory: An analysis of decisions under risk. Econometrica, 47, 313–327. ArticleGoogle Scholar
- Leleur, S., Salling, K. B., Pilkauskiene, I., & Nicolaisen, M. S. (2015). Combining reference class forecasting with overconfidence theory for better risk assessment of transport infrastructure investments. European Journal of Transport and Infrastructure Research, 15(3), 362–375. Google Scholar
- Servranckx, T., Vanhoucke, M., & Aouam, T. (2021). Practical application of reference class forecasting for cost and time estimations: Identifying the properties of similarity. European Journal of Operational Research, 295, 1161–1179. ArticleGoogle Scholar
- Vandevoorde, S., & Vanhoucke, M. (2006). A comparison of different project duration forecasting methods using earned value metrics. International Journal of Project Management, 24, 289–302. ArticleGoogle Scholar
- Vanhoucke, M., & Vandevoorde, S. (2007). A simulation and evaluation of earned value metrics to forecast the project duration. Journal of the Operational Research Society, 58, 1361–1374. ArticleGoogle Scholar
- Walczak, R., & Majchrzak, T. (2018). Implementation of the reference class forecasting method for projects implemented in a chemical industry company. Acta Oeconomica Pragensia, 26(1), 25–33. ArticleGoogle Scholar
Author information
Authors and Affiliations
- Faculty of Economics and Business Administration, Ghent University, Gent, Belgium Mario Vanhoucke
- Mario Vanhoucke